MetEOC-3 provides Metrological uncertainty analysis of OLCI sensor onboard Sentinel-3 satellite
The European Union’s Earth Observation program, Copernicus, provides accurate, timely and easily accessible information regarding our planet and its environment. A key component of the program is the Sentinel family of satellites, developed by the European Space Agency (ESA). There are currently five missions in orbit comprising of 8 Sentinel satellites, which have objectives ranging from measuring sea levels to monitoring air pollution.
One of these missions is Sentinel-3, (see figure 1), which has a primary focus to monitor the ocean and coastal areas. Currently, there are two Sentinel-3 satellites in orbit, with the first, Sentinel-3A, launched in February 2016 followed by Sentinel-3B in April 2018. A key payload carried by Sentinel-3 is the Ocean and Land Colour Instrument (OLCI), an imaging spectrometer made up of 5 cameras (known as detectors), which produces images spanning 1270 km in spectral bands across the visible to near-infrared region of the spectrum. The measurements from OLCI provide data on the nature and health of the biosphere, in particular phytoplankton in the oceans which are responsible for absorbing about a quarter of the carbon dioxide emitted by mankind.
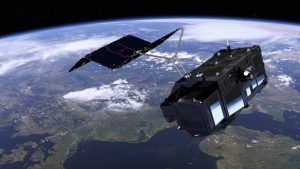
As part of the MetEOC-3 project, an uncertainty analysis of the OLCI radiance product was carried out, following the framework developed in the Fidelity and Uncertainty in Climate Data Records from Earth Observation (FIDUCEO) project. To begin with, the measurement function, which shows how the output product is calculated from the inputs to the sensor, is identified. A schematic representation of the measurement function, known as an ‘uncertainty tree diagram’ is then constructed, see figure 2 (below).
This diagram illustrates the process by which the output is calculated, and allows clear identification of sources of error, while also showing where they are introduced. Using the uncertainty tree, ‘effects tables’ are filled out. These codify the sources of uncertainty which have been identified in the tree, and concisely communicates any correlation between the errors.
The analysis of OLCI served as a means of assessing the best way to provide uncertainties to users, and additionally as a gap analysis. There are a number of corrections that are applied as part of the processing of OLCI images, which include, for example, corrections for light reflected off the instrument falling onto the detector (known as ‘stray light’), and compensation for effects within the detector itself. It was found that these effects were not known is a much detail as we would have liked, so further studies have been proposed to enable a complete analysis.
The radiometric calibration of OLCI, which gives the relationship between the light which falls on to the detector and how this is converted into a digital signal, also presented an interesting problem. In flight it is assessed through observation of a solar illuminated panel within the instrument, and these measurements then feed into a model, which is built up over time. As a result of the model, each new calibration is influenced by all previous calibrations, which creates a complex correlation structure. It was decided that this also needs a further metrological study. Following on from our study of OLCI, it has been proposed that this type of metrological assessment of the instrument should be built in to the development of satellite missions, to aid performance assessment in the future.
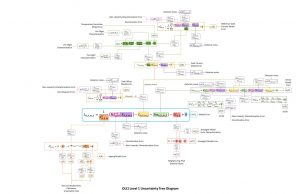